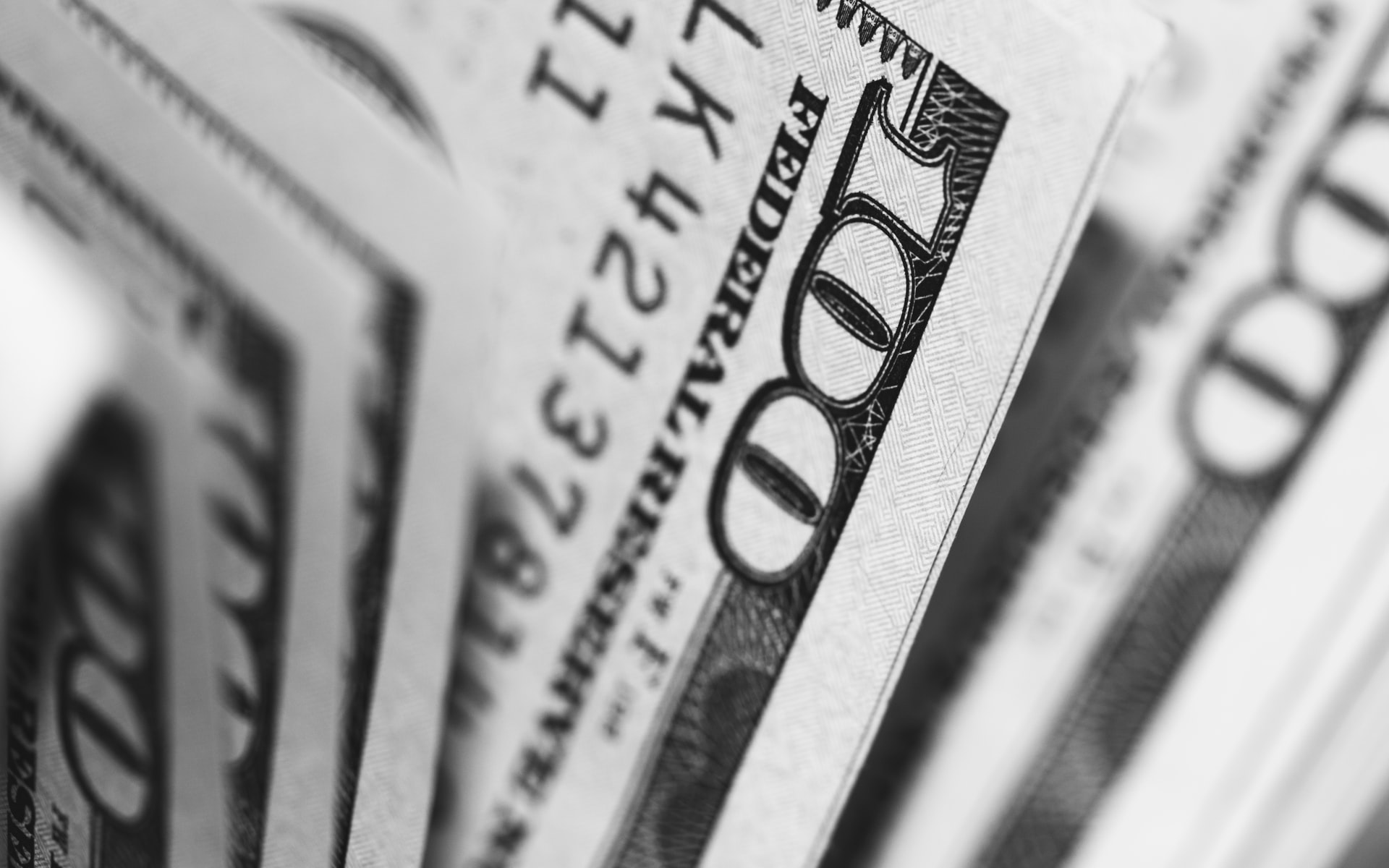
In today’s saturated consumer landscape, the marketing teams that win are the ones that earnestly ask what each customer wants, and relentlessly use data to find the answers. In recent years, analytics and predictive models have enabled forward-thinking marketers to get ahead. But as these techniques get more ubiquitous, the competition is catching up. So what’s next for marketing teams on the cutting-edge?
At Vidora, we believe that uplift modeling is the next big frontier for data-driven marketers. Uplift predictions cut right to the core of what matters to you: how will each customer respond to a marketing campaign? This helps you target scarce resources only where they’re likely to drive ROI, and to deliver marketing experiences that your customers actually want.
You don’t need to know the algorithms – leave those to your data scientists or vendors. But understanding how and when to use uplift models can help supercharge your marketing operation. In this post, we’ll break down everything you need to know about uplift models.
Moving toward prescriptive data
To understand the value of uplift modeling, we first need to examine how it compares to other forms of analysis. When you get past the buzzwords, most data is used in one of three ways: descriptive, predictive, or prescriptive. Uplift models fall into the prescriptive category, where data goes beyond providing information and instead informs what actions you should take to optimize your business.
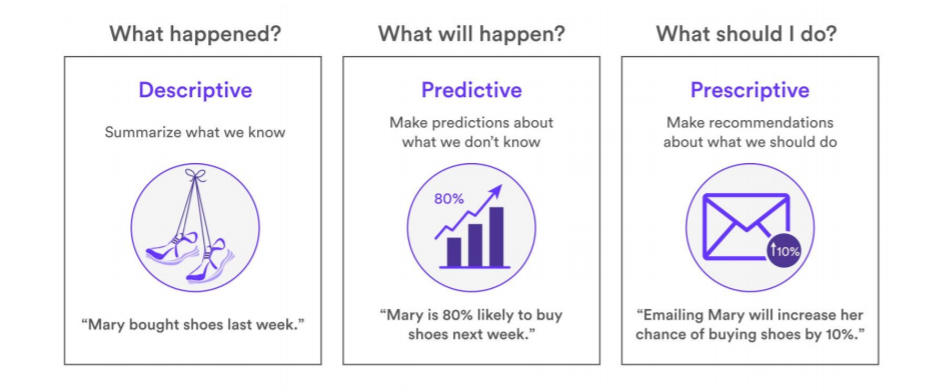
Thanks to well-established analytics providers, most marketers today have access to descriptive data. As marketers get more analytical and technology gets more accessible, usage of predictive modeling is on the rise, too. Both of these approaches are hugely valuable to marketing teams, arming them with precise information about their customers. But if your goal is to influence outcomes rather than to simply understand or predict them, you’re still left to guesswork about what to do with this information. Prescriptive data (and in particular, uplift modeling) bridges that gap. And if you’re already collecting data for descriptive analytics, you’re just one step away from uplift modeling.
What is uplift modeling?
Uplift modeling is a prescriptive technique which predicts how each customer is likely to respond to a marketing action. In other words, uplift predictions tell you which customers you should target in order to maximize the ROI of your campaign.
Defining an uplift prediction involves specifying two inputs:
- Intervention: Which marketing action(s) would you like to measure the impact of?
- Outcome: What is the conversion event that your intervention is meant to influence?
For example, if we are considering offering a discount (the intervention) with the goal of driving customer transactions (the outcome), our uplift prediction for Customer A might tell us: “A discount will increase Customer A’s probability of purchasing by 10% compared to if we did not offer the discount.”
Uplift modeling is still an active area of research in the data science community, but its practical applications are quickly gaining steam in the marketing world. Uber, Wayfair, and Fidelity Investments are among the growing list of companies that rely extensively on uplift modeling.
What are the benefits of an uplift model?
If your aim is to influence customer behavior and you have marketing levers which can meaningfully drive the right outcomes, then uplift is the most effective way to pull those levers. To see why, let’s return to the example above: your team’s goal is to increase transactions by offering some customers a discount.
If we used descriptive analytics to determine our campaign targets, we might create a rule: “offer a discount to any customer who purchased last month”. These customers were willing to purchase in the past, so maybe they’ll buy again. Maybe a discount will nudge them over the line. Maybe. But we’re making two big assumptions – (1) a customer who previously purchased is a prime target to purchase again, and (2) that a discount will be effective in getting that customer to purchase when they otherwise wouldn’t. In reality, some past purchasers may now be uninterested, and communication may only pester them. Others may buy again regardless of the discount, so we’re needlessly cutting into our revenue.
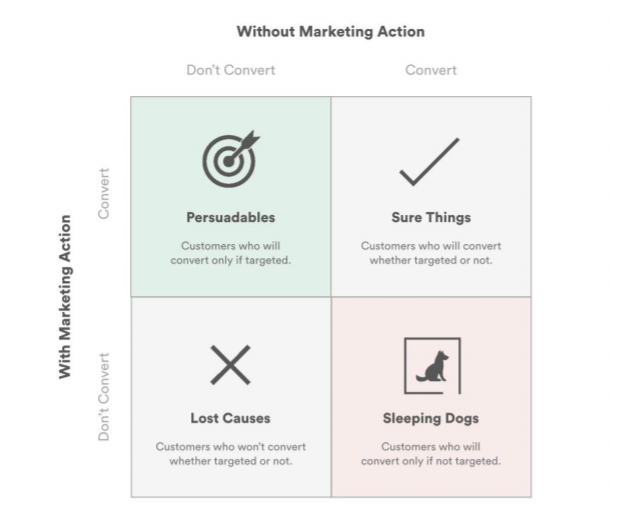
If instead we take a predictive approach and target any customer who is predicted as >70% likely to purchase this month, we’ve eliminated assumption (1). The data is now telling us exactly who may be on the verge of purchasing. But, we’re still assuming that our discount will get those users to purchase.
When we use prescriptive data and uplift modeling, however, we’ve shed all assumptions. The data is telling us exactly whose probability of purchasing will increase because of the discount. Our approach with the data is now aligned with our goal, allowing us to target those who will benefit from a discount without either (a) wasting money on customers who will buy anyway, or (b) over-communicating with uninterested customers.
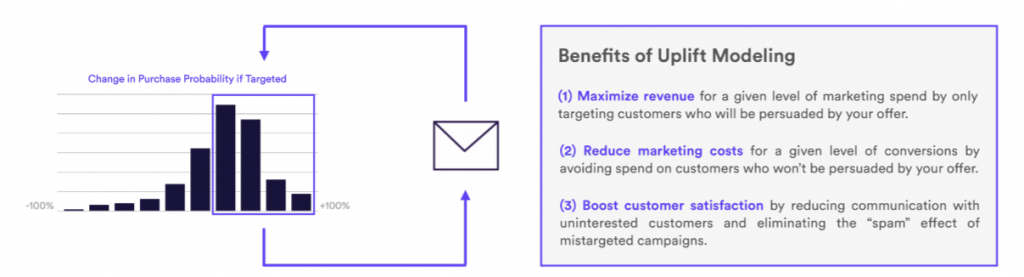
Some real world examples
The advantages of the uplift approach extend to many common marketing problems. A few examples are listed below:
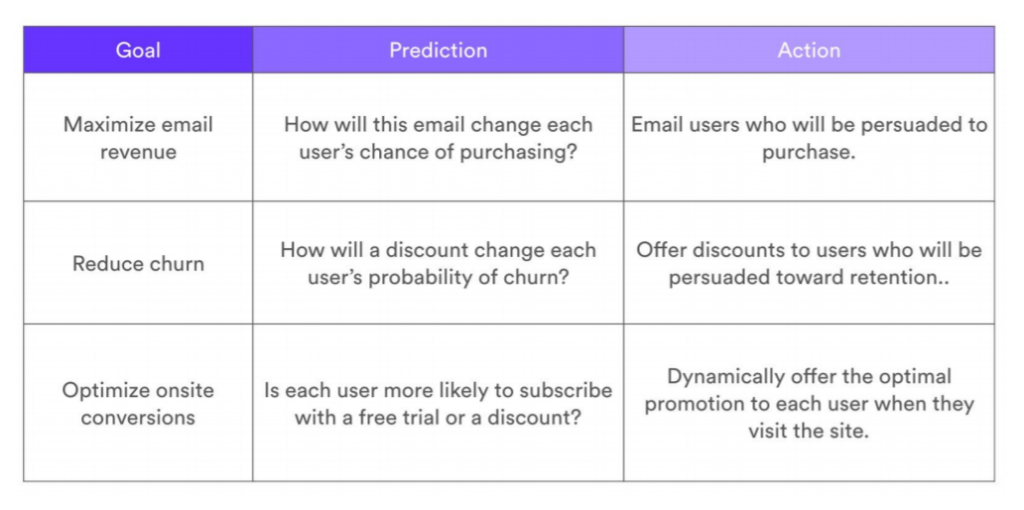
When do uplift models work best?
Uplift modeling can be a game-changer for the right marketing applications. But it’s not a silver bullet. What’s more, building an uplift model requires you to first run an A/B test in which some users are randomly selected to receive the intervention that you’re interested in testing. This added data requirement can be a strain on internal resources, so it’s important to know when the rewards might be worth that extra effort.
Uplift modeling tends to work well when:
- Your goal is to impact short-term user behavior (e.g. a transaction). If instead your goal is to generally strengthen the customer relationship or impact longer term metrics like CLV, uplift may not be up to the task
- You have powerful marketing incentives at your disposal. If your intervention can’t meaningfully sway customer behavior, there may not be any uplift for your model to find.
Uplift modeling and Cortex
At Vidora we are committed to providing a machine learning platform (Cortex) accessible to marketers and product managers. Cortex enables these users to explore the benefits of techniques like uplift modeling without any coding or machine learning experience. What’s more, Cortex integrates directly with both your data sources and your marketing automation tools, facilitating an end-to-end system of automation backed by prescriptive intelligence. Find out more by emailing partners@vidora.com.
Photo by Pepi Stojanovski on Unsplash
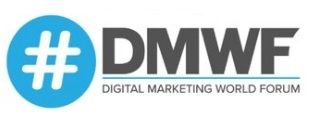
Interested in hearing leading global brands discuss subjects like this in person?
Find out more about Digital Marketing World Forum (#DMWF) Europe, London, North America, and Singapore.